Introducing the Grid Event Signature Library
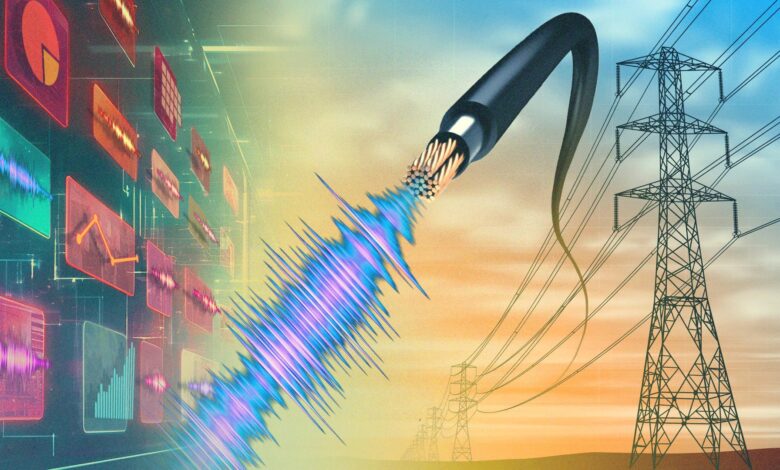
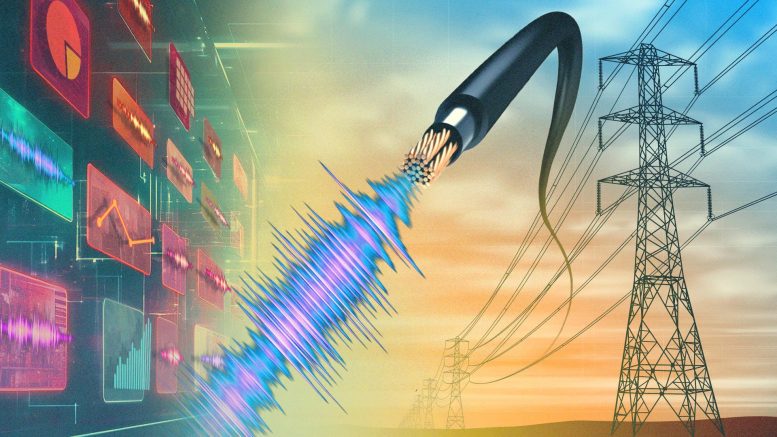
The Grid Signature Event Library energizes utility and researcher understanding of grid behavior by providing access to datasets of waveforms from grid operations. Credit: Adam Malin/ORNL, U.S. Dept. of Energy
Library of operating data enables analysis of complex electric grids.
The Grid Event Signature Library at Oak Ridge National Laboratory offers waveform datasets that help analyze and predict electric grid behaviors. With contributions from various utilities, the library facilitates machine learning models to forecast and mitigate grid malfunctions, enhancing grid reliability and safety.
Introduction to the Grid Event Signature Library
Researchers at Oak Ridge National Laboratory have opened a new virtual library where visitors can check out waveforms instead of books.
The Grid Event Signature Library is an open-access online collection of datasets containing waveforms, which are visual representations of behaviors of the electric grid. Utilities and research institutions contributed anonymized data including voltage, current, and frequency information collected by operating equipment. So far, more than 350 users worldwide have utilized the library, which provides a vital understanding of an increasingly complex grid.
Enhancing Grid Management Through Advanced Data Analysis
“Researchers can search different variables, like the type of sensor or a description of the event – for example, a waveform that represents a blown transformer and its effect on transmission line voltage,” said ORNL’s Aaron Wilson, who manages the tool.
A recent paper described the datasets and their applications. Machine learning can be trained to recognize waveforms that provide an early warning of equipment malfunction, enabling power system operators to prevent blackouts, wildfires, and damage to the power grid.
Reference: “The Grid Event Signature Library: An Open-Access Repository of Power System Measurement Signatures” by Aaron J. Wilson, Ali Riza Ekti, Jim Follum, Shuchismita Biswas, Christabella Annalicia, Jhi-Young Joo, Omer Aziz and Jamie Lian, 24 May 2024, IEEE.
DOI: 10.1109/ACCESS.2024.3404886