How the Brain Masters Complexity Without Descending Into Chaos
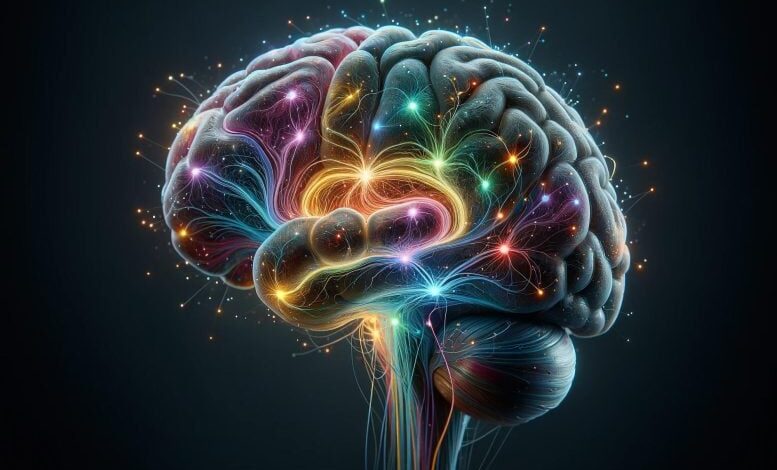
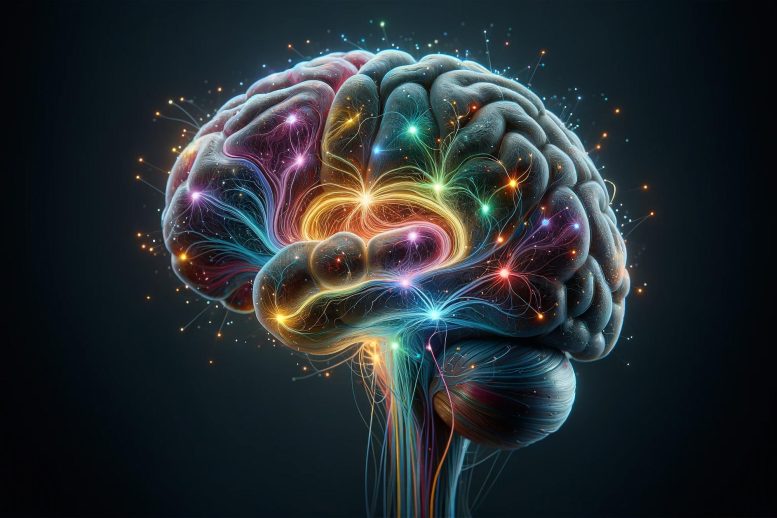
A recent study in Neuron details how neurons with “mixed selectivity” empower our brains to manage multiple computations simultaneously, enhancing cognitive flexibility. This capability allows neurons, particularly in the medial prefrontal cortex, to participate in a variety of mental tasks, boosting both cognitive capacity and creativity. The study also discusses mechanisms such as oscillations and neuromodulators that focus these neurons on relevant tasks, underscoring the vital role of mixed selectivity in brain functionality. Credit: SciTechDaily.com
Researchers reveal that neurons exhibiting “mixed selectivity” enable our brains to handle multiple computations simultaneously, providing the flexibility needed for complex cognitive tasks.
Many neurons exhibit “mixed selectivity,” meaning they can integrate multiple inputs and participate in multiple computations. Mechanisms such as oscillations and neuromodulators recruit their participation and tune them to focus on the relevant information.
Every day our brains strive to optimize a trade-off: With lots of things happening around us even as we also harbor many internal drives and memories, somehow our thoughts must be flexible yet focused enough to guide everything we have to do. In a new paper published in the journal Neuron, a team of neuroscientists describes how the brain achieves the cognitive capacity to incorporate all the information that’s relevant without becoming overwhelmed by what’s not.
The Role of Mixed Selectivity
The authors argue that the flexibility arises from a key property observed in many neurons: “mixed selectivity.” While many neuroscientists used to think each cell had just one dedicated function, more recent evidence has shown that many neurons can instead participate in a variety of computational ensembles, each working in parallel. In other words, when a rabbit considers nibbling on some lettuce in a garden, a single neuron might be involved in not only assessing how hungry it feels but also whether it can hear a hawk overhead or smell a coyote in the trees and how far away the lettuce is.
The brain does not multitask, said paper co-author Earl K. Miller, Picower Professor in The Picower Institute for Learning and Memory at MIT and a pioneer of the mixed selectivity idea, but many cells do have the capacity to be roped into multiple computational efforts (essentially “thoughts”). In the new paper, the authors describe specific mechanisms the brain employs to recruit neurons into different computations and to ensure that those neurons represent the right number of dimensions of a complex task.
“These neurons wear multiple hats,” Miller said. “With mixed selectivity you can have a representational space that’s as complex as it needs to be and no more complex. That’s what flexible cognition is all about.”
Co-author Kay Tye, Professor at The Salk Institute and the University of California at San Diego, said mixed selectivity among neurons particularly in the medial prefrontal cortex is key to enabling many mental abilities.
“The mPFC is like a hum of whispers that represents so much information through highly flexible and dynamic ensembles,” Tye said. “Mixed selectivity is the property that endows us with our flexibility, cognitive capacity, and ability to be creative. It is the secret to maximizing computational power which is essentially the underpinnings of intelligence.”
Origins of an Idea
The idea of mixed selectivity germinated in 2000 when Miller and colleague John Duncan defended a surprising result from a study of cognition in Miller’s lab. As animals sorted images into categories, about 30 percent of the neurons in the prefrontal cortex of the brain seemed to be involved. Skeptics who believed that every neuron had a dedicated function scoffed that the brain would devote so many cells to just one task. Miller and Duncan’s answer was that perhaps cells had the flexibility to be involved in many computations. The ability to serve on one cerebral task force, as it were, did not preclude them from being able to serve many others.
But what benefit does mixed selectivity convey? In 2013 Miller teamed up with two co-authors of the new paper, Mattia Rigotti of IBM Research and Stefano Fusi of Columbia University, to show how mixed selectivity endows the brain with powerful computational flexibility. Essentially, an ensemble of neurons with mixed selectivity can accommodate many more dimensions of information about a task than a population of neurons with invariant functions.
“Since our original work, we’ve made progress understanding the theory of mixed selectivity through the lens of classical machine learning ideas,” Rigotti said. “On the other hand, questions dear to experimentalists about the mechanisms implementing it at a cellular level had been comparatively under-explored. This collaboration and this new paper set out to fill that gap.”
In the new paper the authors imagine a mouse who is considering whether to eat a berry. It might smell delicious (that’s one dimension). It might be poisonous (that’s another). Yet another dimension or two of the problem could come in the form of a social cue. If the mouse smells the berry scent on a fellow mouse’s breath, then the berry is probably OK to eat (depending on the apparent health of the fellow mouse). A neural ensemble with mixed selectivity would be able to integrate all that.
Recruiting Neurons
While mixed selectivity has the backing of copious evidence—it has been observed across the cortex and in other brain areas such as the hippocampus and amygdala—there are still open questions. For instance, how are neurons recruited to tasks and how do neurons that are so “open-minded” remain tuned only to what really matters to the mission?
In the new study, the researchers who also include Marcus Benna of UC San Diego and Felix Taschbach of The Salk Institute, define the forms of mixed selectivity that researchers have observed, and argue that when oscillations (also known as “brain waves”) and neuromodulators (chemicals such as serotonin or dopamine that influence neural function) recruit neurons into computational ensembles, they also help them “gate” what’s important for that purpose.
To be sure, some neurons are dedicated to a specific input, but the authors note they are an exception rather than the rule. The authors say these cells have “pure selectivity.” They only care if the rabbit sees lettuce. Some neurons exhibit “linear mixed selectivity,” which means their response predictably depends on multiple inputs adding up (the rabbit sees lettuce and feels hungry). The neurons that add the most dimensional flexibility are the “nonlinear mixed selectivity” ones that can account for multiple independent variables without necessarily summing them. Instead they might weigh a whole set of independent conditions (e.g. there’s lettuce, I’m hungry, I hear no hawks, I smell no coyotes, but the lettuce is far and I see a pretty sturdy fence).
So what brings neurons into the fold to focus on the salient factors, however many there are? One mechanism is oscillations, which are produced in the brain when many neurons all maintain their electrical activity at the same rhythm. This coordinated activity enables information sharing, essentially tuning them together like a bunch of cars all playing the same radio station (maybe the broadcast is about a hawk circling overhead). Another mechanism the authors highlight is neuromodulators. These are chemicals that upon reaching receptors within cells can influence their activity as well. A burst of acetylcholine, for instance, might similarly attune neurons with the right receptors to certain activity or information (like maybe that feeling of hunger).
“These two mechanisms likely work together to dynamically form functional networks,” the authors write.
Understanding mixed selectivity, they continue, is critical to understanding cognition.
“Mixed selectivity is ubiquitous,” they conclude. “It is present across species and across functions from high-level cognition to ‘automatic’ sensorimotor processes such as object recognition. The widespread presence of mixed selectivity underscores its fundamental role in providing the brain with the scalable processing power needed for complex thought and action.”
Reference: “Mixed selectivity: Cellular computations for complexity” by Kay M. Tye, Earl K. Miller, Felix H. Taschbach, Marcus K. Benna, Mattia Rigotti and Stefano Fusi, 9 May 2024, Neuron.
DOI: 10.1016/j.neuron.2024.04.017